In what follows a variety of techniques will be used on the same problem to provide a better feel. ML models are often called black-box models because they allow a pre-set number of empty parameters or nodes to be assigned values by the machine learning algorithm.
What Is A Black Box In Machine Learning Quora
With the abundance of well-documented machine learning ML libraries its fairly straightforward for a.
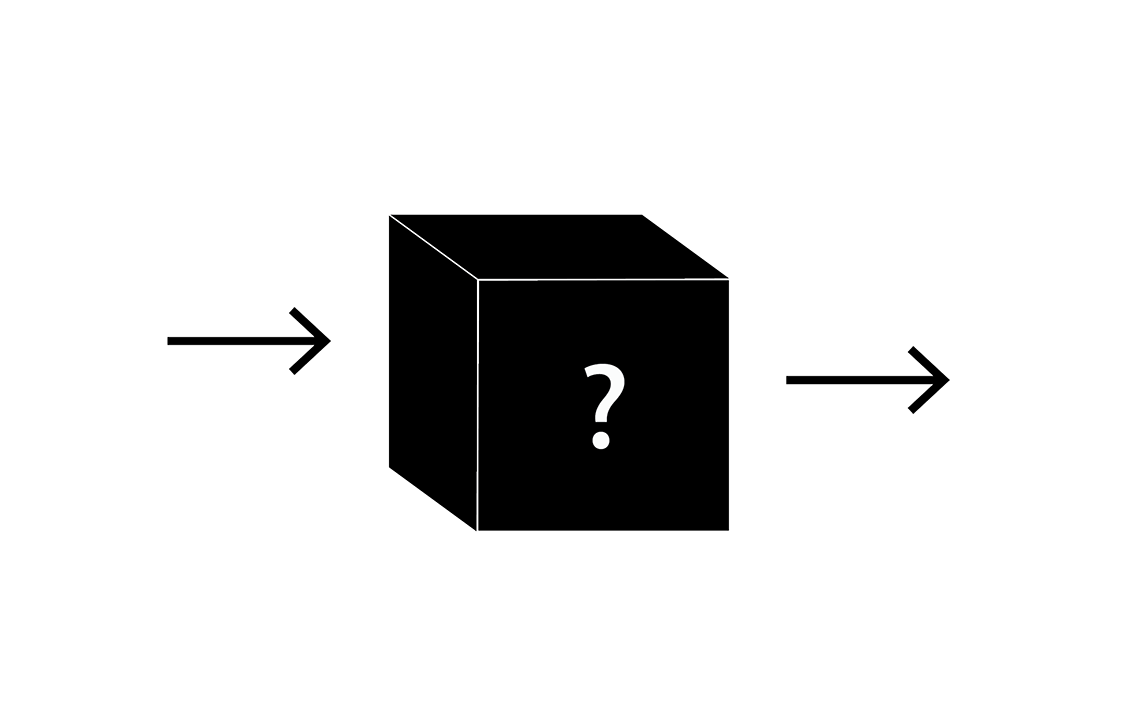
Black box machine learning. From black boxes to white boxes - Mihaela van der Schaar - YouTube. An Important Introduction to Interpretable Machine Learning Models in Python. The black box in Artificial Intelligence AI or Machine Learning programs 1 has taken on the opposite meaning.
But the systems operate as black boxes meaning their selection techniques are hidden from users. The answer to that question is yes. In the simplest case a machine learning model can be a linear regression and consist of a line defined by an explicit algebraic equation.
Recently developed automated machine-learning AutoML systems iteratively test and modify algorithms and those hyperparameters and select the best-suited models. Machine learning is frequently referred to as a black boxdata goes in decisions come out but the processes between input and output are opaque. And a lot of the work done in the field involves developing techniques that try to explain the decision made by a machine learning algorithm without breaking open the black box.
Opening the Black Box Its now time to see some machine learning in action. AbstractFriends Dont Let Friends Deploy Black Box Models. In the near future the transformative potential of machine learning could revolutionise areas such as.
The latest approach in Machine Learning where there have been important empirical successes 2 is Deep Learning yet there are significant concerns about transparency. The increased attention to black-box machine learning has given rise to a body of research on explainable AI. This is not a black box method since it is clear how the variables are being used to compute an output.
Because of model complexity their inner logic is not readily intelligible to a human hence the common critique of ML models as black boxes. In machine learning often a trade off must be made between accuracy and intelligibility. Particularly for neural networks where input data can undergo complex transformations in multiple layers of the algorithm the model can become vastly complex and behave in unpredictable ways.
If it is too complex to understand and explain then it is a black box whether or not we can calculate the results or not. Therefore users may not trust the results and can find it difficult to tailor the systems to their search needs. Decoding the Black Box.
Machine learning ML has emerged as a powerful tool for harnessing big biological data. Black Box Machine Learning - YouTube. Rosenberg Bloomberg ML EDU September 20 2017 26 67.
Specifically the back-propagation step is responsible for updating the weights based on its error function. Can there be machine learning without black boxes. Even if one has a list of the input variables black box predictive models can be such complicated functions of the variables that no human can understand how the variables.
In machine learning these black box models are created directly from data by an algorithm meaning that humans even those who design them cannot understand how variables are being combined to make predictions. In an especially egregious circumstance of poor guesswork that came to light during the 2018 California wildfires Google used a proprietary black-box machine-learning algorithm from another company to power its search page weather widget. The complex structure underlying ML models can potentially provide insights into the problems they are used to solve.
Machine Learning can be rightly considered Black boxes solutions for the XOR problem using neural networks can be modelled but as the number of inputs grow so does the complexity and dimensions.